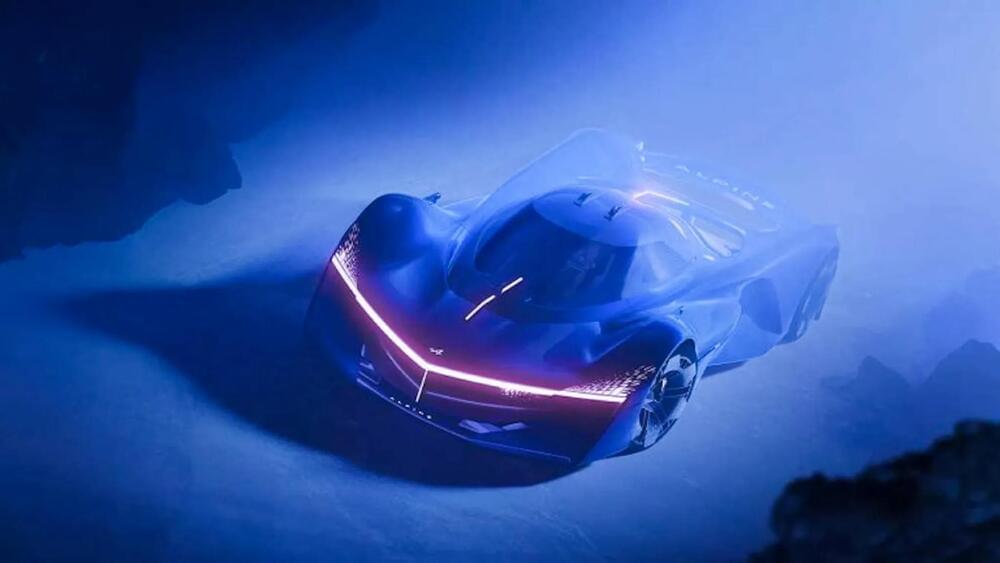
In a bold move towards sustainability in the automotive industry, Alpine has introduced its new V6 hydrogen engine. The engine is a groundbreaking development that merges high-performance engineering with eco-friendly technology. This innovative engine represents a significant leap for the French automotive brand, showcasing its commitment to advancing hydrogen as a viable fuel alternative in the world of motorsport and beyond.
While Japanese automobile company Toyota continues to be leading the hydrogen revolution, other automobile companies are following closely behind. While some have placed all their bets on electric vehicles being the future of sustainable engines, others are looking at ways to continue producing the internal combustion engine. The answer may be found in hydrogen technology whereby traditional internal combustion engines can be adapted to support the alternative fuel source.
Alpine previously introduced a hydrogen powered car in 2022. Now, the newer model is twice as powerful as the last. The car features a 3.5-litre, twin-turbo V6 engine. It produces a power output of 740bhp, and can reach up to 9,000rpm with 770 Nm of torque. The two hydrogen engines are located in the sidepods and behind the cockpit. The model has been in the works for two years and is a testament to Alpine’s continued dedication towards sustainability.