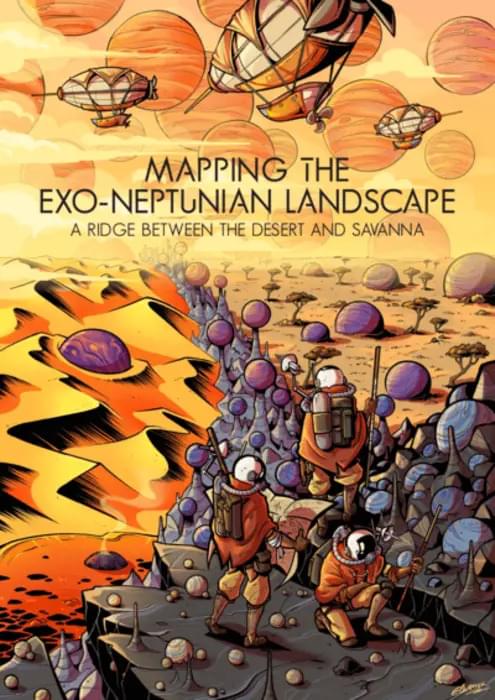
How do the characteristics of Neptune-like exoplanets, also known as exo-Neptunes, differ from each other? This is what a recent study published in Astronomy and Astrophysics hopes to address as an international team of researchers investigated a new classification known as the “Neptunian Ridge”. This complements previous classifications of “Neptunian Desert” and “Neptunian Savannah”, with the former identifying exo-Neptunes that are rare in number but orbit very close to their parent stars while the “Neptune Savannah” describes exo-Neptunes that orbit much farther out. This study holds the potential to help astronomers better understand the formation and evolution of exo-Neptunes throughout the cosmos.
For the study, the researchers used confirmed and candidate exoplanets that comprise the Kepler DR25 catalog to ascertain the characteristic variations in exo-Neptunes while providing additional insights into the formation and evolution of exo-Neptunes, as well. In the end, they determined that this “Neptunian Ridge” exists as a middle-ground between the “Neptunian Desert” and “Neptunian Savannah”, with the former hypothesized to have formed from moving inward in their system from high-eccentricity tidal migration and the latter forming from disk-driven migration, which occurs right after planetary formation.
“Our work to observe this new structure in space is highly significant in helping us map the exoplanet landscape,” said Dr. David Armstrong, who is an Associate Professor of Physics at the University of Warwick and a co-author on the study. “As scientists, we’re always striving to understand why planets are in the condition they are in, and how they ended up where they are. The discovery of the Neptunian ridge helps answer these questions, unveiling part of the geography of exoplanets out there, and is a hugely exciting discovery.”