Will Humans Have to Merge with AI to Survive?
What if the only way to survive the AI revolution is to stop being human?
Ray Kurzweil, one of the most influential futurists and the godfather of AI, predicts that humans will soon reach a turning point where merging with AI becomes essential for survival. But what does this truly mean? Will we evolve into superintelligent beings, or will we lose what makes us human?
In this video, we explore Kurzweil’s bold predictions, the concept of the Singularity, and the reality of AI-human integration. From Neuralink to the idea of becoming “human cyborgs,” we examine whether merging with AI is an inevitable step in human evolution—or a path toward losing our biological identity.
Are we truly ready for a world where there are no biological limitations?
Chapters:
Intro 00:00 — 01:11
Ray Kurzweil’s Predictions 01:11 — 02:23
Singularity Is Nearer 02:23 — 04:05
What Does “Merging with AI” Really Mean? 04:05 — 04:35
Neuralink 04:35 — 07:02
Why Would We Need to Merge with AI? 07:02 — 10:04
Human Life After Merging with AI 10:04 — 12:30
Idea of Becoming ‘Human Cyborg’ 12:30 — 14:33
No Biological Limitations 14:33 — 17:24
#RayKurzweil #AI #Singularity #HumanCyborg #FutureTech #ArtificialIntelligence
Category: robotics/AI – Page 148
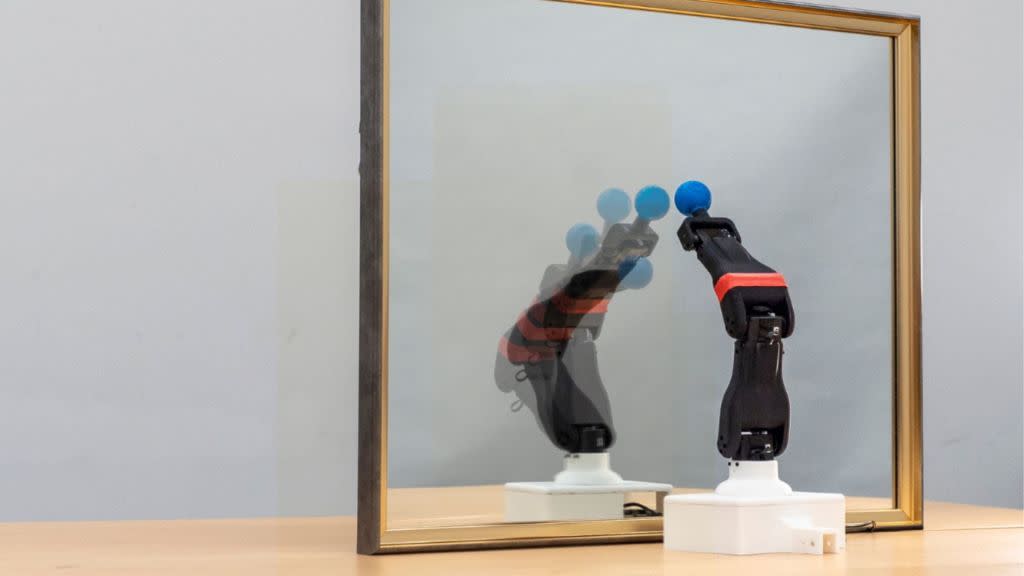
Robot Uses Mirror Reflection to Perfect Movements and Self-Repair
Humans naturally perceive their bodies and anticipate movement outcomes, a trait robotic experts aim to replicate in machines for enhanced adaptability and efficiency.
Now, researchers have developed an autonomous robotic arm capable of learning its physical form and movement by observing itself through a camera. This approach is akin to a robot learning to dance by watching its reflection.
Columbia Engineering researchers claim this technique enables robots to adapt to damage and acquire new skills autonomously.
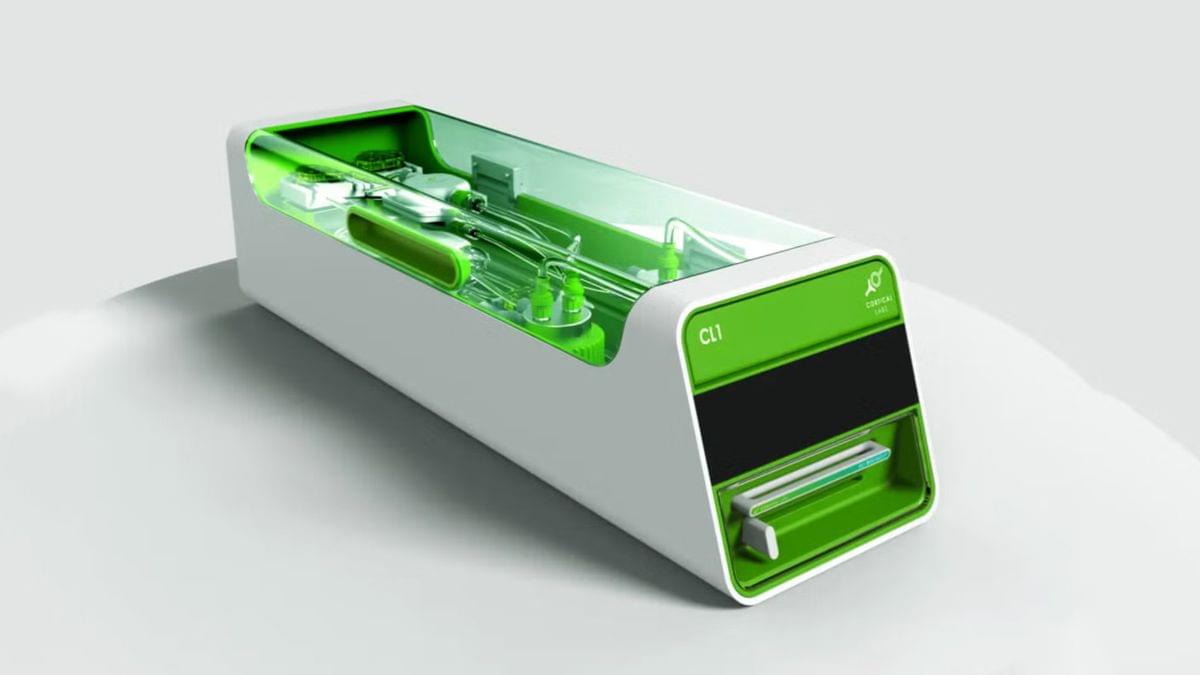
World’s first ‘body in a box’ biological computer uses human brain cells with silicon-based computing
A notable aspect of the CL1 is its ability to learn and adapt to tasks. Previous research has demonstrated that neuron-based systems can be trained to perform basic functions, such as playing simple video games. Cortical Labs’ work suggests that integrating biological elements into computing could improve efficiency in tasks that traditional AI struggles with, such as pattern recognition and decision-making in unpredictable environments.
Cortical Labs says that the first CL1 computers will be available for shipment to customers in June, with each unit priced at approximately $35,000.
The use of human neurons in computing raises questions about the future of AI development. Biological computers like the CL1 could provide advantages over conventional AI models, particularly in terms of learning efficiency and energy consumption. The adaptability of neurons could lead to improvements in robotics, automation, and complex data analysis.
OpenAI’s ChatGPT App On macOS Can Now Directly Edit Code
ChatGPT, OpenAI’s AI-powered chatbot platform, can now directly edit code — if you’re on macOS, that is. The newest version of the ChatGPT app for macOS can take action to edit code in supported developer tools, including Xcode, VS Code, and JetBrains. Users can optionally turn on an auto-apply mode so ChatGPT can make edits without the need for additional clicks.
Subscribers to ChatGPT Plus, Pro, and Team can use the code editing feature as of Thursday by updating their macOS app. OpenAI says that code editing will roll out to Enterprise, Edu, and free users next week.
In a post on X, Alexander Embiricos, a member of OpenAI’s product staff working on desktop software, added that the ChatGPT app for Windows will get direct code editing “soon.”
Direct code editing builds on OpenAI’s work with apps ChatGPT capability, which the company launched in beta in November 2024. Work with apps allows the ChatGPT app for macOS to read code in a handful of dev-focused coding environments, minimizing the need to copy and paste code into ChatGPT.
With the ability to directly edit code, ChatGPT now competes more directly with popular AI coding tools like Cursor and GitHub Copilot. OpenAI reportedly has ambitions to launch a dedicated product to support software engineering in the months ahead.
AI coding assistants are becoming wildly popular, with the vast majority of respondents in GitHub’s latest poll saying that they’ve adopted AI tools in some form. Y Combinator partner Jared Friedman recently claimed a quarter of YC’s W25 startup batch have 95% of their codebases generated by AI.
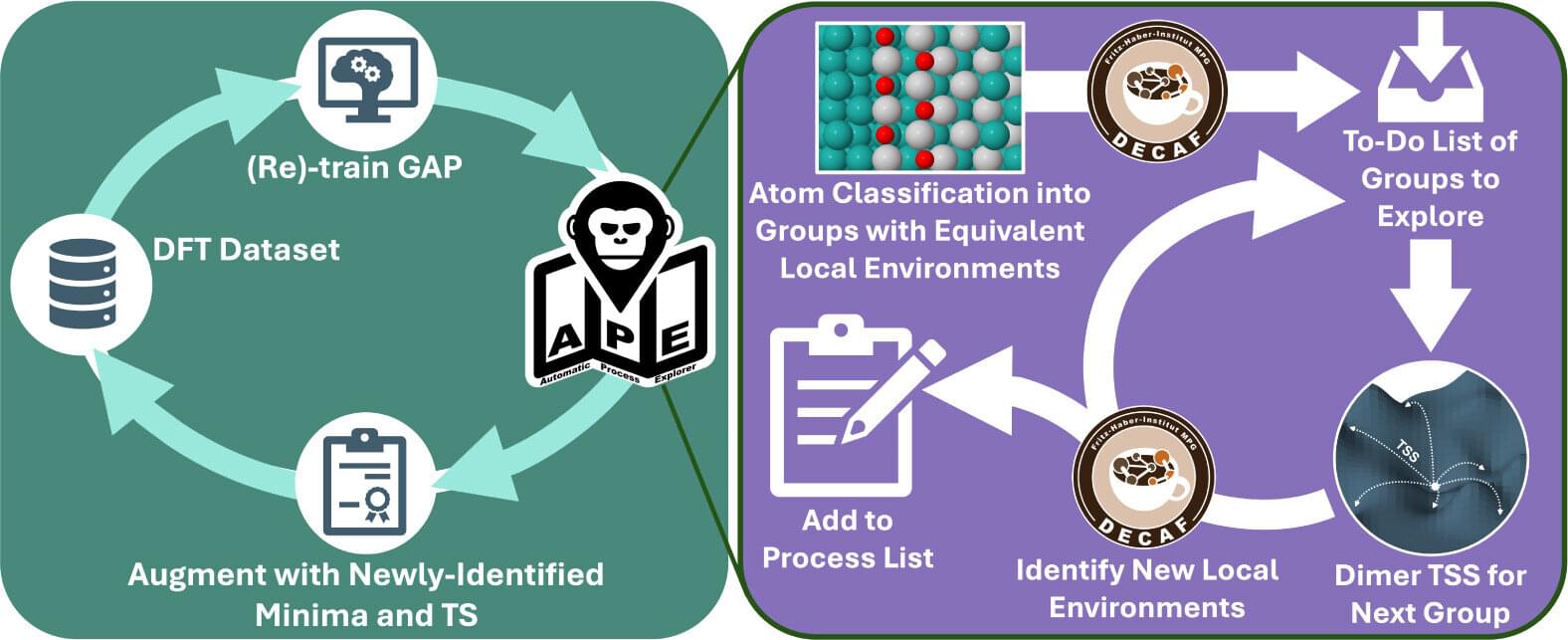
Machine learning reveals hidden complexities in palladium oxidation, sheds light on catalyst behavior
Researchers at the Fritz Haber Institute have developed the Automatic Process Explorer (APE), an approach that enhances our understanding of atomic and molecular processes. By dynamically refining simulations, APE has uncovered unexpected complexities in the oxidation of palladium (Pd) surfaces, offering new insights into catalyst behavior. The study is published in the journal Physical Review Letters.
Kinetic Monte Carlo (kMC) simulations are essential for studying the long-term evolution of atomic and molecular processes. They are widely used in fields like surface catalysis, where reactions on material surfaces are crucial for developing efficient catalysts that accelerate reactions in energy production and pollution control. Traditional kMC simulations rely on predefined inputs, which can limit their ability to capture complex atomic movements. This is where the Automatic Process Explorer (APE) comes in.
Developed by the Theory Department at the Fritz Haber Institute, APE overcomes biases in traditional kMC simulations by dynamically updating the list of processes based on the system’s current state. This approach encourages exploration of new structures, promoting diversity and efficiency in structural exploration. APE separates process exploration from kMC simulations, using fuzzy machine-learning classification to identify distinct atomic environments. This allows for a broader exploration of potential atomic movements.
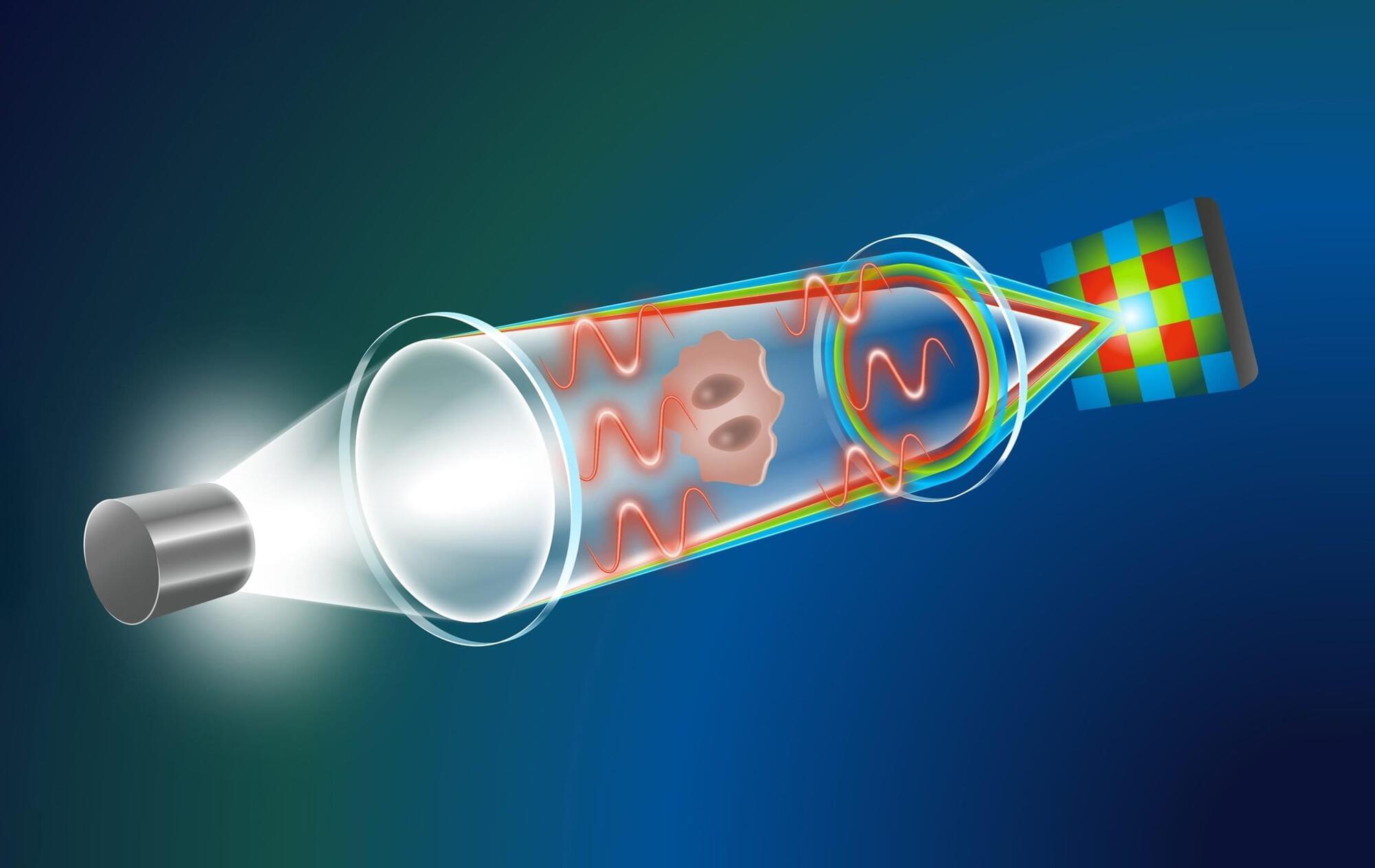
Scientists Just Discovered a Hidden Superpower in Microscopes — Thanks to AI
Traditional microscopy often relies on labeling samples with dyes, but this process is costly and time-consuming. To overcome these limitations, researchers have developed a computational quantitative phase imaging (QPI) method using chromatic aberration and generative AI.
By leveraging the natural variations in focus distances of different wavelengths, the technique constructs through-focus image stacks from a single exposure. With the help of a specially trained diffusion model, this approach enables high-quality imaging of biological specimens, including real-world clinical samples like red blood cells. The breakthrough could revolutionize diagnostics, providing an accessible and efficient alternative to conventional imaging techniques.
Revealing Insights Without Labels
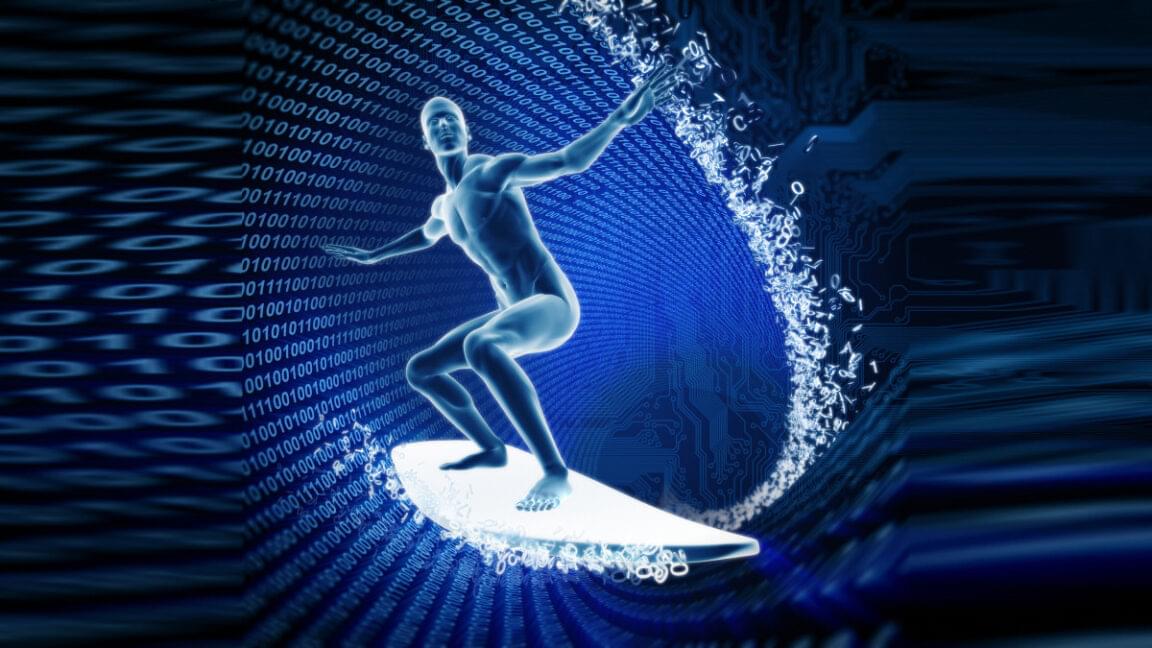
James Giordano ASO
The future of warfare starts in your mind. Understand how Neuroscience, Technology/AI and the OODA loop affects your flow. The world is changing, and cognitive warfare is at the forefront. In our latest podcast episode, we sit down with James Giordano, PhD, a Navy veteran and an expert in neurocognitive science, to delve into the world of cognitive warfare.
Stay in the Loop: https://www.aglx.com/newsletter-signup-north-america.
From the impact of emotions on decision-making to the integration of artificial intelligence and human cognition, this episode challenges your perspective on the battlefield. Join us as we explore the ethical implications of genetic modifications, the transformative effects of psychedelics, and the complexities of data usage in the digital age. Get ready to reimagine the relationship between technology, culture, and language. Don’t miss out on this opportunity to gain valuable insights from our thought-provoking conversation with Dr. Giordano. Tune in now to stay ahead of the curve on the evolving landscape of warfare!
00:00 — Understanding the OODA loop: A Neuroscience Perspective.
09:11 — Exploring Fifth Generation Warfare and Liminal Warfare.
16:06 — The Long Game: China’s Strategic Plan.
22:19 — Understanding Cognitive Warfare and Human-Machine Teaming.
25:52 — The Evolution of Human-Machine Teaming.
29:11 — Human Involvement in AI Decision Making.
36:01 — The Ethics of Paternalistic AI Systems.
40:43 — Technology’s Impact on Cognitive Engagement.
45:13 — Exploring Technologies for Human Performance Enhancement.
55:59 — Diving Into Attacking Mode and Ethics.
56:24 — Hacking the Human Genome.
59:37 — Epigenetic Modification and Phenotypic Shift.
1:04:54 — The Psychedelic Revolution.
1:11:18 — Revisiting Alcohol and Caffeine: Benefits and Burdens.
1:19:18 — Impact of Technology on Cognitive Capacity.
1:23:33 — Information Overload and Burdens.
1:27:02 — Ownership and Security of Personal Data.
1:31:56 — Identifying Predispositional Traits.
1:33:49 — Data Manipulation and Biometrics.
1:40:13 — Cultural Impact of Technology.
1:48:55 — The Role of Education in Integrating Science, Technology, Ethics, and Policy.
1:54:30 — Major Threats and Concerns in Today’s World.
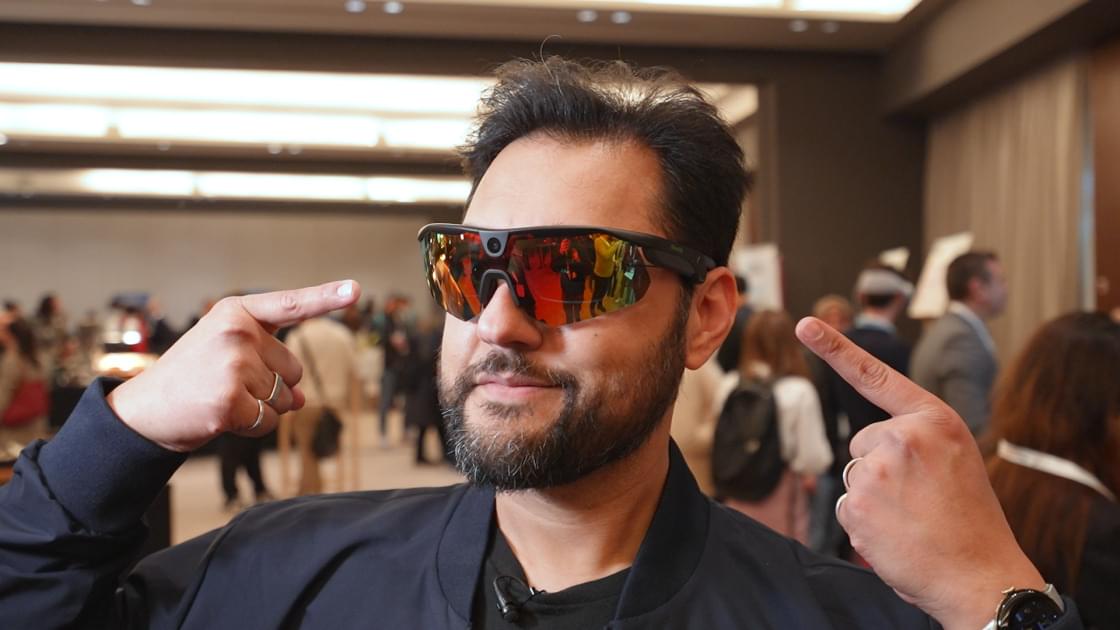
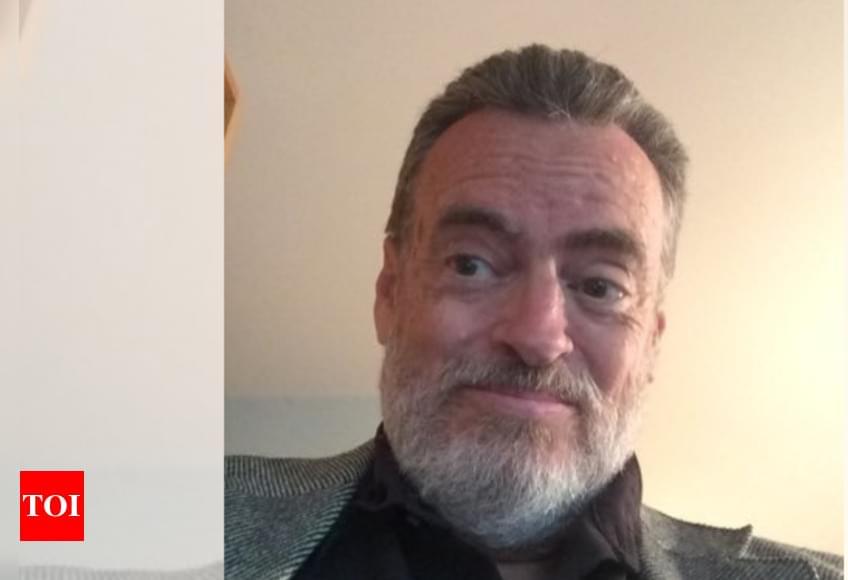