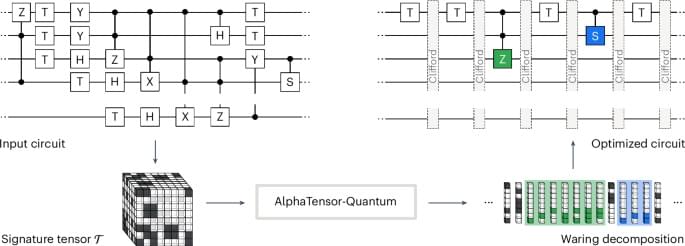
AlphaTensor–Quantum addresses three main challenges that go beyond the capabilities of AlphaTensor25 when applied to this problem. First, it optimizes the symmetric (rather than the standard) tensor rank; this is achieved by modifying the RL environment and actions to provide symmetric (Waring) decompositions of the tensor, which has the beneficial side effect of reducing the action search space. Second, AlphaTensor–Quantum scales up to large tensor sizes, which is a requirement as the size of the tensor corresponds directly to the number of qubits in the circuit to be optimized; this is achieved by a neural network architecture featuring symmetrization layers. Third, AlphaTensor–Quantum leverages domain knowledge that falls outside of the tensor decomposition framework; this is achieved by incorporating gadgets (constructions that can save T gates by using auxiliary ancilla qubits) through an efficient procedure embedded in the RL environment.
We demonstrate that AlphaTensor–Quantum is a powerful method for finding efficient quantum circuits. On a benchmark of arithmetic primitives, it outperforms all existing methods for T-count optimization, especially when allowed to leverage domain knowledge. For multiplication in finite fields, an operation with application in cryptography34, AlphaTensor–Quantum finds an efficient quantum algorithm with the same complexity as the classical Karatsuba method35. This is the most efficient quantum algorithm for multiplication on finite fields reported so far (naive translations of classical algorithms introduce overhead36,37 due to the reversible nature of quantum computations). We also optimize quantum primitives for other relevant problems, ranging from arithmetic computations used, for example, in Shor’s algorithm38, to Hamiltonian simulation in quantum chemistry, for example, iron–molybdenum cofactor (FeMoco) simulation39,40. AlphaTensor–Quantum recovers the best-known hand-designed solutions, demonstrating that it can effectively optimize circuits of interest in a fully automated way. We envision that this approach can accelerate discoveries in quantum computation as it saves the numerous hours of research invested in the design of optimized circuits.
AlphaTensor–Quantum can effectively exploit the domain knowledge (provided in the form of gadgets with state-of-the-art magic-state factories12), finding constructions with lower T-count. Because of its flexibility, AlphaTensor–Quantum can be readily extended in multiple ways, for example, by considering complexity metrics other than the T-count such as the cost of two-qubit Clifford gates or the qubit topology, by allowing circuit approximations, or by incorporating new domain knowledge. We expect that AlphaTensor–Quantum will become instrumental in automatic circuit optimization with new advancements in quantum computing.