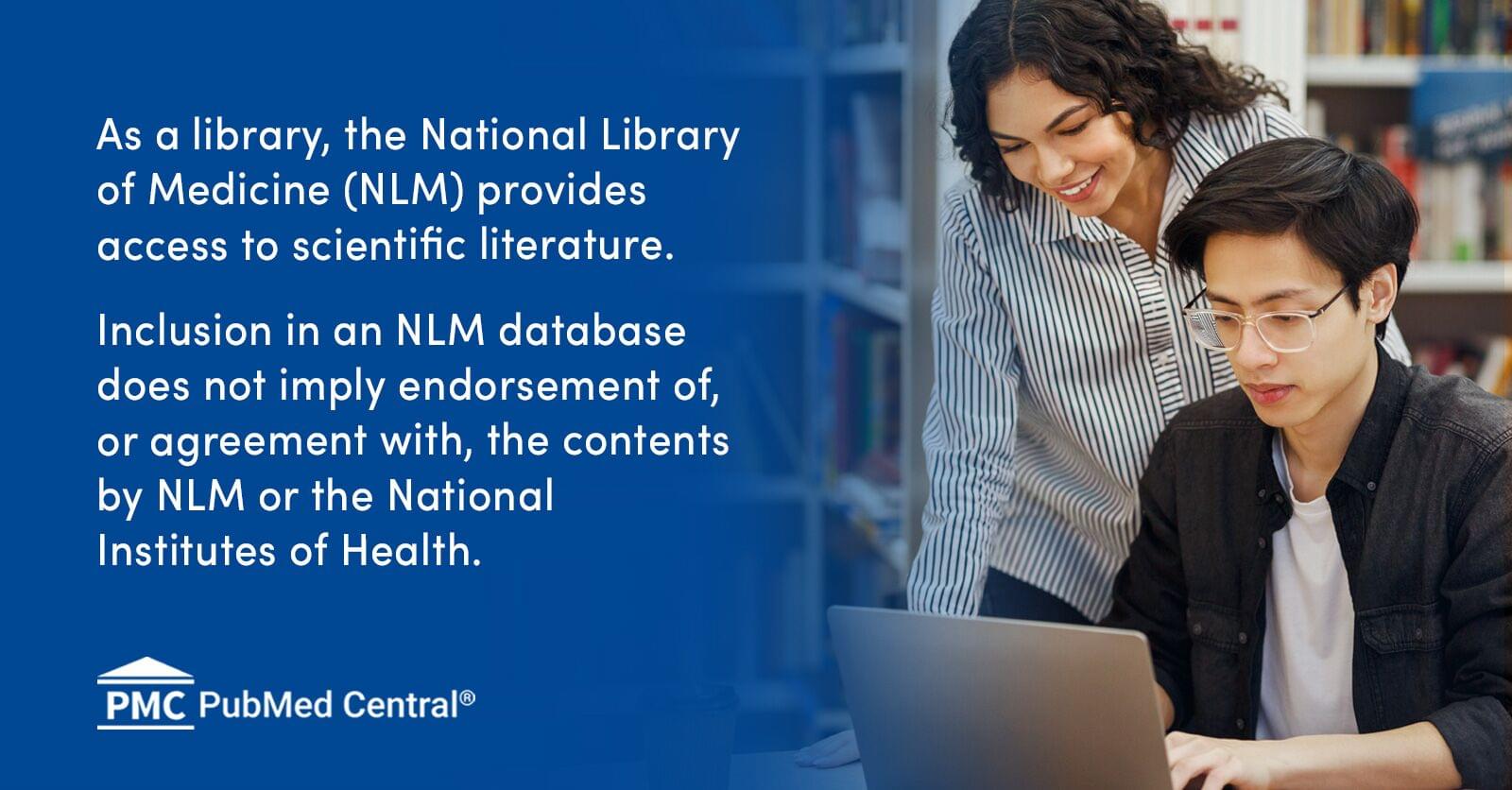
The healthcare industry faces a significant shift towards digital health technology, with a growing demand for real-time and continuous health monitoring and disease diagnostics [1, 2, 3]. The rising prevalence of chronic diseases, such as diabetes, heart disease, and cancer, coupled with an aging population, has increased the need for remote and continuous health monitoring [4, 5, 6, 7]. This has led to the emergence of artificial intelligence (AI)-based wearable sensors that can collect, analyze, and transmit real-time health data to healthcare providers so that they can make efficient decisions based on patient data. Therefore, wearable sensors have become increasingly popular due to their ability to provide a non-invasive and convenient means of monitoring patient health. These wearable sensors can track various health parameters, such as heart rate, blood pressure, oxygen saturation, skin temperature, physical activity levels, sleep patterns, and biochemical markers, such as glucose, cortisol, lactates, electrolytes, and pH and environmental parameters [1, 8, 9, 10]. Wearable health technology includes first-generation wearable technologies, such as fitness trackers, smartwatches, and current wearable sensors, and is a powerful tool in addressing healthcare challenges [2].
The data collected by wearable sensors can be analyzed using machine learning (ML) and AI algorithms to provide insights into an individual’s health status, enabling early detection of health issues and the provision of personalized healthcare [6,11]. One of the most significant advantages of AI-based wearable health technology is to promote preventive healthcare. This enables individuals and healthcare providers to proactively address symptomatic conditions before they become more severe [12,13,14,15]. Wearable devices can also encourage healthy behavior by providing incentives, reminders, and feedback to individuals, such as staying active, hydrating, eating healthily, and maintaining a healthy lifestyle by measuring hydration biomarkers and nutrients.