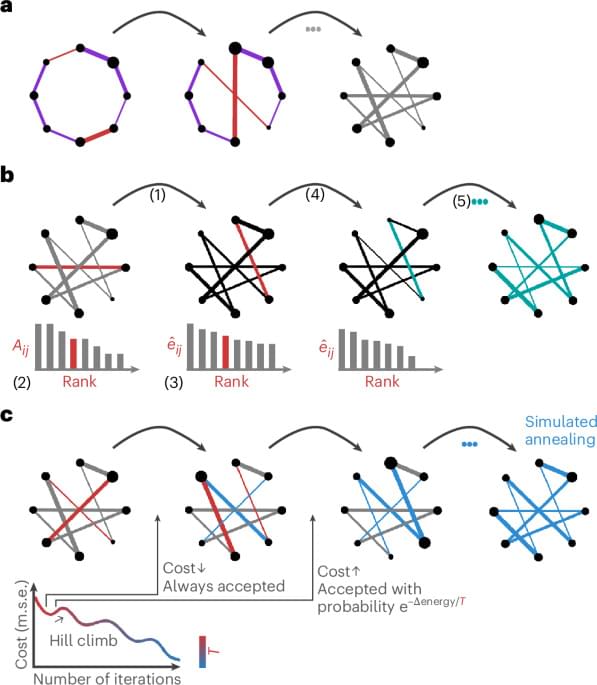
While we have established, using rank-based methods, that the simulated annealing algorithm outperforms other randomization techniques in preserving the empirical network’s strength sequence, we have not quantified how well the different models preserve the strength distribution. The level to which the empirical strength distribution is preserved in a null network is crucial, because it ensures an accurate representation of influential graph features, such as hubs, whose importance is intricately tied to characteristics of the distribution.
To assess the goodness of fit between the strength distributions of the empirical and the randomized structural networks, we superimpose their cumulative distribution functions (Fig. 2b and Supplementary Fig. 8). Across all datasets, the curves produced via simulated annealing show the best match to the empirical strength cumulative distribution function with almost perfect superposition. Furthermore, the curves obtained using the Rubinov–Sporns and the Maslov–Sneppen algorithms show considerably more variability across null networks as shown by their wider spread, recapitulating previously observed patterns of underestimation and overestimation across datasets (see ‘Null model calibration’ section in Supplementary Information). To confirm these observations quantitatively, we compute Kolmogorov–Smirnov test statistics between the cumulative strength distributions of the empirical and each randomized network, measuring the maximum distance between them (Fig. 2b and Supplementary Fig. 8). Across all datasets, the simulated annealing algorithm outperforms the other two null models with significantly lower Kolmogorov–Smirnov statistics (P ≈ 0, CLES of 100% for all two-tailed, Wilcoxon–Mann–Whitney two-sample rank-sum tests). Furthermore, in the HCP dataset and the higher resolution Lausanne network, the Rubinov–Sporns algorithm generated cumulative strength distributions with slightly worse correspondence to the empirical distribution than the cumulative strength distributions yielded by the Maslov–Sneppen algorithm (LAU, high resolution: P 10−176, CLES of 61.58%; HCP: P ≈ 0, CLES of 100% for all empirical networks, two-tailed, Wilcoxon–Mann–Whitney two-sample rank-sum test).
As an illustration, we consider whether the nulls generated by different algorithms recapitulate fundamental characteristics associated with the empirical strength distribution. Namely, we focus on the heavy tailedness of the strength distribution (that is, does the null network also have a heavy-tailed strength distribution, suggesting the presence of hubs?) and the spatial location of high-strength hub nodes. We assess heavy tailedness and identify hubs using the nonparametric procedure outlined in refs. 73,74 (see Methods for more details).