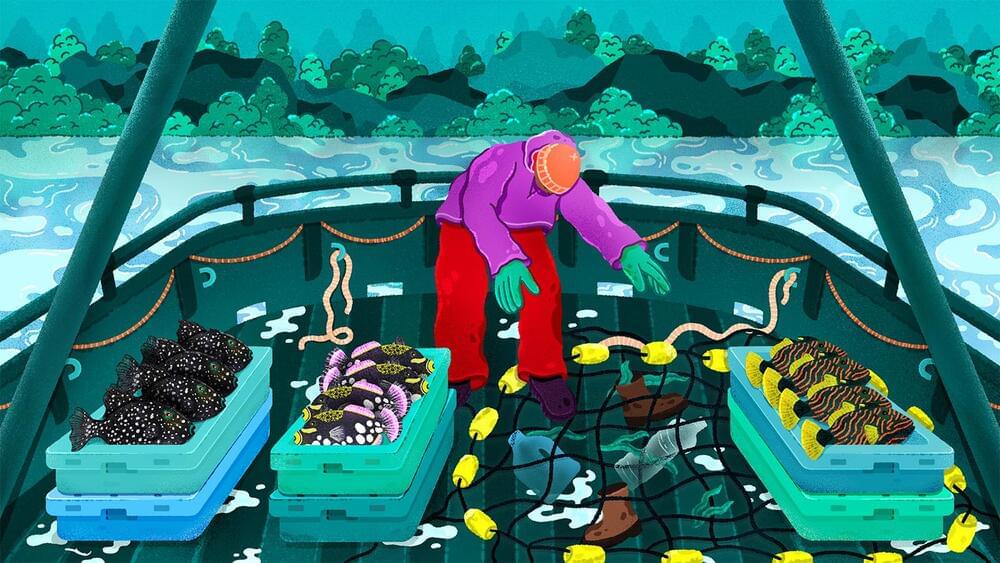
In 2010, Mike Williams traveled from London to Amsterdam for a physics workshop. Everyone there was abuzz with the possibilities—and possible drawbacks—of machine learning, which Williams had recently proposed incorporating into the LHCb experiment. Williams, now a professor of physics and leader of an experimental group at the Massachusetts Institute of Technology, left the workshop motivated to make it work.
LHCb is one of the four main experiments at the Large Hadron Collider at CERN. Every second, inside the detectors for each of those experiments, proton beams cross 40 million times, generating hundreds of millions of proton collisions, each of which produces an array of particles flying off in different directions. Williams wanted to use machine learning to improve LHCb’s trigger system, a set of decision-making algorithms programmed to recognize and save only collisions that display interesting signals—and discard the rest.
Of the 40 million crossings, or events, that happen each second in the ATLAS and CMS detectors—the two largest particle detectors at the LHC—data from only a few thousand are saved, says Tae Min Hong, an associate professor of physics and astronomy at the University of Pittsburgh and a member of the ATLAS collaboration. “Our job in the trigger system is to never throw away anything that could be important,” he says.